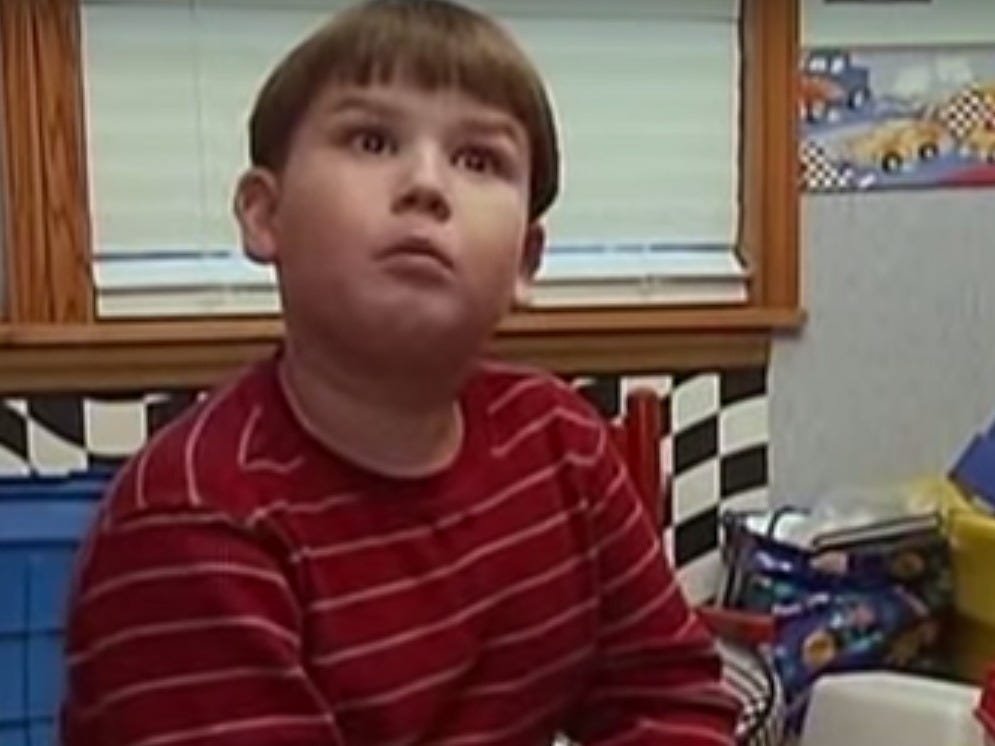
the overfitting pardox: too much of a good thing
Ronan Patrick
02/04/2020
CHARLES GOODHART FAMOUSLY SAID: “WHEN A MEASURE BECOMES A TARGET, IT CEASES TO BE A GOOD MEASURE.” OVERFITTING, DATA STRATEGIST RONAN PATRICK ARGUES, IS THE REASON WHY… AND THE AD INDUSTRY IS RIDDLED WITH IT.
BACON IS GOOD FOR ME
In the primetime television series US Wife Swap, ‘King’ Curtis reacts to the binning of his bacon and chicken nuggets with the iconic line: “bacon is good for me.”
‘King’ Curtis, waving his flabby arms up and down like a mating penguin, protests that the things he’s not meant to eat taste so good, whilst the vegetables he’s meant to eat taste like soggy cardboard.
He has a point.
The answer is that our taste buds are a benchmark used to measure health. Fat, sugar and salt were vitally rare nutrients, so for hundreds of thousands of years being drawn to them was an effective measure for a sustaining diet.
Being able to modify the foods available to us broke that dynamic. We can now add fat and sugar to foods and avoid the vegetables and high-fibre carbs that previously formed 90% of our diet. Our taste buds have become a tainted chalice that invite us to drink from the poison within.
The advertising industry is full of King Curtises. Full of wonks who regularly fall prey to a problem computer scientists have dubbed ‘overfitting.’
In computer science, the question of how to create benchmarks that allow a program to learn from past experience is at the heart of machine learning, and the cause of poor performance is often a failure to tread the delicate tight-rope between underfitting and overfitting.
Simply put, a program that fails because it doesn’t adhere strictly enough to the benchmarks provided is ‘underfit’. If, by contrast, a program fails because it is too sensitive to the particular data points, then that is overfitting.
You will have seen overfitting everywhere.
If ‘King’ Curtis decides that life consists of more than chicken nuggets and bacon, he may decide to go to the gym. Certain visible signs of fitness, low body fat and high muscle mass are associated with a healthy body and lower risk of disease. But these too are proxy benchmarks. One could overfit the signals, becoming bulimic to reduce body fat or taking steroids to increase muscle mass to give you the picture of good health… but only the picture.
Or perhaps ‘King’ Curtis takes up a sport, say, fencing. The original goal of fencing was to teach duelling, hence the name, defencing. The benchmark created to establish one’s fencing prowess came in the form of a button on the tip of the blade that register hits. But techniques that would serve you poorly in a real duel can trigger the tip. Modern fencers now use blades as flexible as possible that allow them to flick the blade at their opponent, grazing just hard enough to score.
Were a modern fencer to duel a Napoleonic cavalry officer to the death, the former would probably be cut to shreds as they attempted to spank their adversary with their wobbly sword.
We often try to perfectly hone our model to the data at hand, but if the data available is in any way flawed, we risk overfitting. Overfitting poses a major danger when we are dealing with noise or mismeasurement… and we almost always are because:
1. There are errors in how the data was collected, or how it was reported.
2. The phenomena we are trying to measure are hard to define, never mind measure.
If we had copious data, completely mistake free and representing exactly what we are trying to evaluate, then yes, overfitting wouldn’t be a concern. But this isn’t the case. Benchmarks are usually a proxy for the thing we really want to measure… but can’t.
Enter the Advertising Industry and its squealing bandwagon of King Curtises.
In an ideal world, our industry would possess a perfect measurement of attribution, that is, we would know that for every purchase of X, what factors nudged that person’s brain to make that purchase.
But we don’t live in an ideal world. Good luck trying to measure attribution for that 51 year-old who, having always wanted one since their teenage years but only recently having made it, splashes out on a BMW.
Cookies enable marketers to track web browsing history prior to purchase and have often been held up as enabling perfect attribution measurements. But in the wake of the Cambridge Analytica scandal, a host of privacy measures have inhibited digital attribution. What’s more, the incoming cookiepocolypse will make attribution modelling even more difficult.
More importantly, though, cookies never explained why that person started googling BMWs in the first place. Was it because they’ve always wanted one since a teenager or because of a 2011 superbowl ad or because they believe it gives them a free license to be an utter wazzock on the roads?
And this assumes that there even is a single clear cause of the purchase. Like the snowball that triggers an avalanche due to months of mounting snow pressure, human decision making is often driven by a host of causes which, once piled on top of each other, become sufficient to drive a purchase.
We don’t know for certain – and that doubt is key. We do have benchmarks, but like the examples at the start of this piece, they are proxy metrics. There’s nothing wrong with proxy metrics, as long as we accept that the benchmarks aren’t perfect.
The Bible warns its followers not to bow down to “any likeness of anything that is in heaven.” This industry has its own idol problem: the idolatry of data. It’s an industry that drools at the feat of benchmarks that are an imperfect likeness of perfect attribution, and then makes poor decisions because of it. I have attempted to capture some of my favourites in the table below:
How do we tell the difference between an excellent advertising campaign and an overfit one?
How do we tell a genuine star performer from a fraud who has overfit their work to the performance metrics?
The primary method computer scientists use to check for overfitting is cross-validation. Say you have 10 key benchmarks which an AI is set to learn from. We might hold back 2 points at random, and fit the model only to the other 8. We then see how well the program has generalised to the two other data points that had not been provided. The two held back points function as canaries in the coal mine: if the complex model nails the 8 training points but wildly misses the 2 test points, it’s a good bet that overfitting is at work.
To put it in English, imagine you have a school which uses standardised tests to measure their students. The way to check whether teachers are genuinely improving their students’ intellectual capacity each year, rather than teaching to the test (overfitting) – you take a random sample of students each and test them with a different evaluation method, perhaps an essay or an oral exam. The secondary data points serve to check if the students are actually improving rather than getting better at test taking.
And no, machine learning in business will not solve all our problems. I’m not pulling a Gary V and using X piece of tech to proclaim the death of something. I’m arguing the precise opposite: there will never be a completely perfect benchmark so we should never give absolute primacy to one system. We need a plurality of measurements, and a healthy dose of scepticism, to correct overfitting.
The implications of these learnings for the advertising industry are worrying, as the concept of cross-validating measurements doesn’t exist in many marketing circles. Indeed, a concerning trend is occurring; as marketing budgets are cut, brands are deciding that the first thing to cut back are the more expensive, rigorous post-campaign measurements.
After all, why do we need to do an expensive econometric study or a Nielsen PCA when we’ve got free social metrics like engagement rate and impressions from social media pages?
But if a brand doesn’t have a strong sense of which marketing activities are having a good or bad effect, how on earth will it identify the stronger campaigns, nevermind incentivise them?
Steve Jobs put it more simply: “incentives are very powerful… so you have to be careful about what you incent people to do.” A company will build whatever the CEO decides to measure.
Best make certain those measurements are cross-validated, because if not, you’ll get a rash of overfitting.
Bibliography
Brian Christian and Tom Griffiths. Algorithms to Live By: The Computer Science of Human Decisions. 2016.